Precision genome engineering
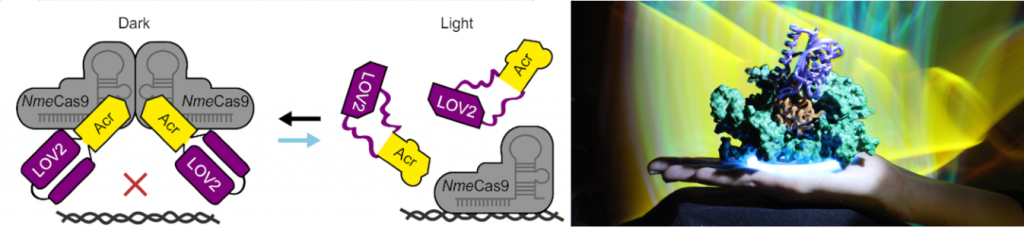
CRISPR systems hold enormous promise for a wide range of genome engineering applications in research, biotechnology and therapy. To improve the precision and safety of CRISPR genome editing, we continuously develop and improve molecular tools to control the activity of CRISPR effectors using exogenous and endogenous stimuli.
Towards these goals, our lab conceived several CRISPR control strategies based on so-called anti-CRISPR proteins (Acrs). Acrs are naturally occurring, potent protein inhibitors of CRISPR effectors. In one research branch, we engineer light-switchable derivatives of natural anti-CRISPRs by embedding photosensor domains, such as the light-oxygen-voltage 2 (LOV2) domain from Avena sativa, into Acr structures [Bubeck & Hoffmann et al., Nat. Methods, 2018; Hoffmann and Mathony, Nucleic Acids Research, 2021]. The resulting, chimeric inhibitors block Cas9 in the dark, but release its activity upon blue light irradiation. We are currently extending this approach to a variety of CRISPR-Cas systems as well as alternative stimuli including clinically approved drugs.
In complementary work, we develop optogenetic CRISPR-deadCas9-based transcriptional activators for controlling the activity of endogenous genes in a light-off mode of operation [Münch et al., Biorxiv, 2023].
In addition to controlling CRISPR with exogenous stimuli, we also harness gene circuit-based logics to facilitate cell-type and tissue-specific genome engineering. In one approach, we link tissue-specific microRNAs to the activity of CRISPR-Cas effectors using microRNA-dependent anti-CRISPR transgenes as mediators. This approach enables, for instance, hepatocyte and cardiomyocyte selective genome editing [Hoffmann et al., Nucleic Acids Research 2019].
Finally, we aim at improving the sequence specificity of CRISPR effectors using protein engineering and computational modeling strategies [Aschenbrenner et al. Science Advances 2020].
Computational design and Machine Learning

Our group is increasingly applying computational modeling approaches and model interpretation strategies to target complex protein engineering problems. In particular, we are applying machine learning techniques at various scales, from relatively simple models (e.g. gradient boosting classifiers) to large language models, dependent on the research goals and datasets available for model training.
In early work, we employed convolutional neural networks to “learn” the relation between protein primary sequence and protein function. Applying model sensitivity analysis based on partial input occlusion then enabled us to identify regions in proteins that are critical for specific protein functions as well as sites amenable to protein engineering [Upmeier zu Belzen et al., Nature Machine Intelligence, 2019].
Currently, our computational efforts mostly focus on the question of how to identify sites suitable for protein domain fusion (i.e. receptor insertion) in effector protein sequences as well as allosteric hotspots and pathways. For model training, we develop our own tailor-made data sets, that we assemble on the basis of public data as well as our own experimental data derived from Flow-seq high-throughput screens [Mathony et al., Advanced science, 2023] and directed evolution experiments.
In collaboration with Bruno Correia’s lab at EPFL, Lausanne, we are also applying structure-centric design approaches, e.g. to improve the effectiveness of CRISPR inhibitory proteins [Mathony et al., Nature Chemical Biology, 2020] since several years. The emergence of DeepMind’s Alphafold and other recent models for protein structure prediction from protein sequence increasingly fuels these exciting efforts.
For model prototyping and training of simple models, we have a GPU workstation available directly in our lab. For resource-intense machine learning tasks, we access the powerful BWfor helix compute infrastructure available for free to academic institutions in the state of Baden-Wuerttemberg.
Engineering Protein Allostery
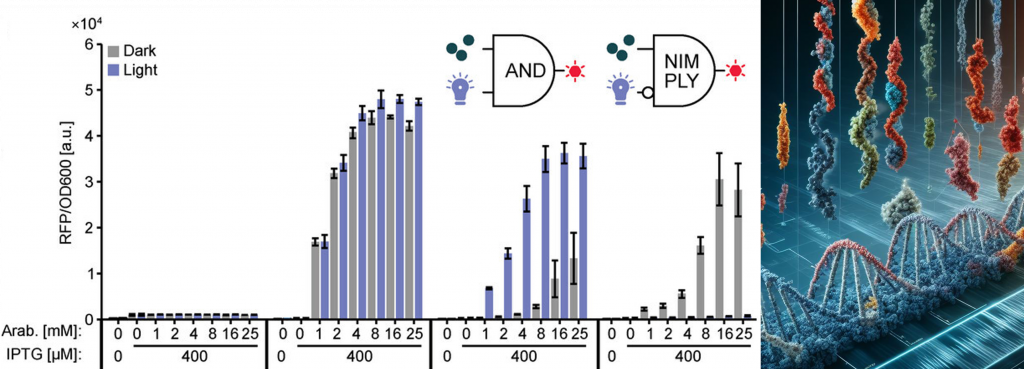
Protein Allostery was once called “the second secret of life” by Monod. And rightly so: Allostery is an extremely effective and elegant principle, by which cells regulate the activity of their molecular machinery and all complex live forms make use of it. From a synthetic biology perspective, being able to effectively engineer allostery is equal to the ability of remotely controlling and orchestrating protein activity and thus to direct functions in living cells and organisms. While Allostery is a well-known regulatory principle that has been intensively studied since decades, it remains very challenging to effectively engineer allosteric regulation [Mathony & Niopek, Advanced Biology, 2021].
The vision of the “DaVinci-Switches” ERC project is to develop experimental methods for the design of switchable, allosteric proteins that can be activated or deactivated remotely via external stimuli. Therefore, we use protein domain interbreeding strategies to create hybrids between protein effectors that execute a certain cellular function and receptor domains capable of sensing a certain stimulus. Using in vivo directed protein evolution strategies as well as high-throughput screening, we eventually create non-natural, allosteric proteins. These can on the one hand be directly applied, but also serve as representative test-cases to train computer models with the aim to eventually rationalize the protein engineering process (see here).
In a recent proof-of-concept study, we screened thousands of protein hybrids created by inserting receptor domains at every possible position of E. coli effector proteins in high-throughput. Thereby, we identified various novel, light-switchable variants of the widely used E. coli transcription factor AraC [Mathony et al., Advanced science, 2023] and deciphered biophysical constraints underlying successful domain interbreeding. We are currently expanding this approach towards a variety of effector proteins widely used in synthetic biology, including transcriptional regulators and CRISPR effectors of various kinds. Further down the road, we intend to place mammalian transcription factors involved in cell reprogramming and differentiation processes under control of exogenous stimuli, which we believe will open up various, exciting applications in regenerative medicine.